Causality. Simplified.
Our state-of-the-art causal reasoning platform is singularly focused on transforming complex, often opaque data into clear, actionable knowledge. Whether you’re a researcher working through intricate datasets, a business leader making pivotal decisions, or a policymaker shaping the future, our causal inference platform is crafted to illuminate the path ahead.
Correlation without causation
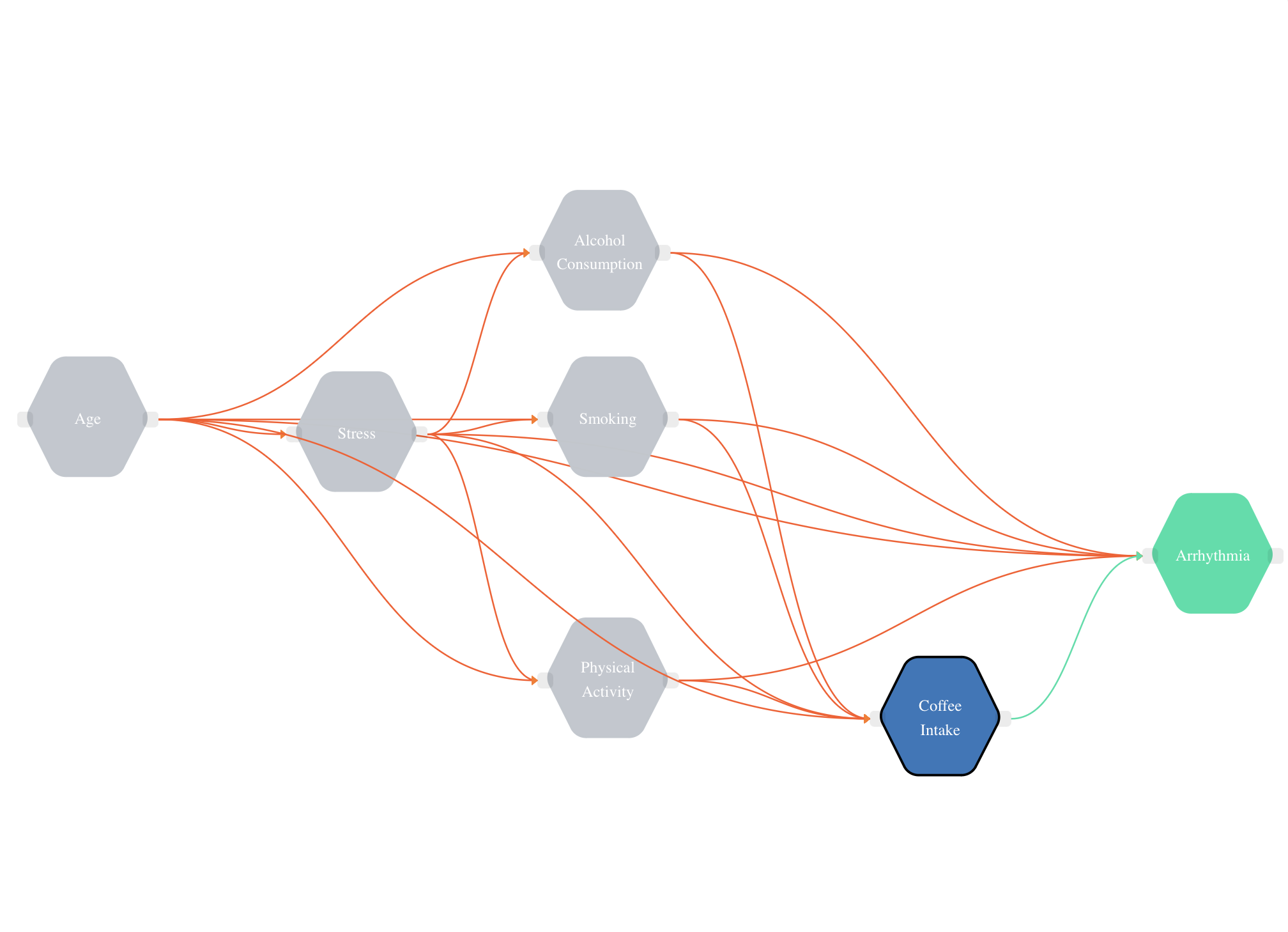
Setting: You found a strong correlation between coffee intake and cardiac arrhythmias. Is this relationship causal? What does the graph tell us? There are several common causes of coffee intake and arrythmia. When this happens, spurious associations flow between coffee intake and arrythmias through the orange paths, distorting our estimate of the causal relationship.
Causation via common cause adjustment
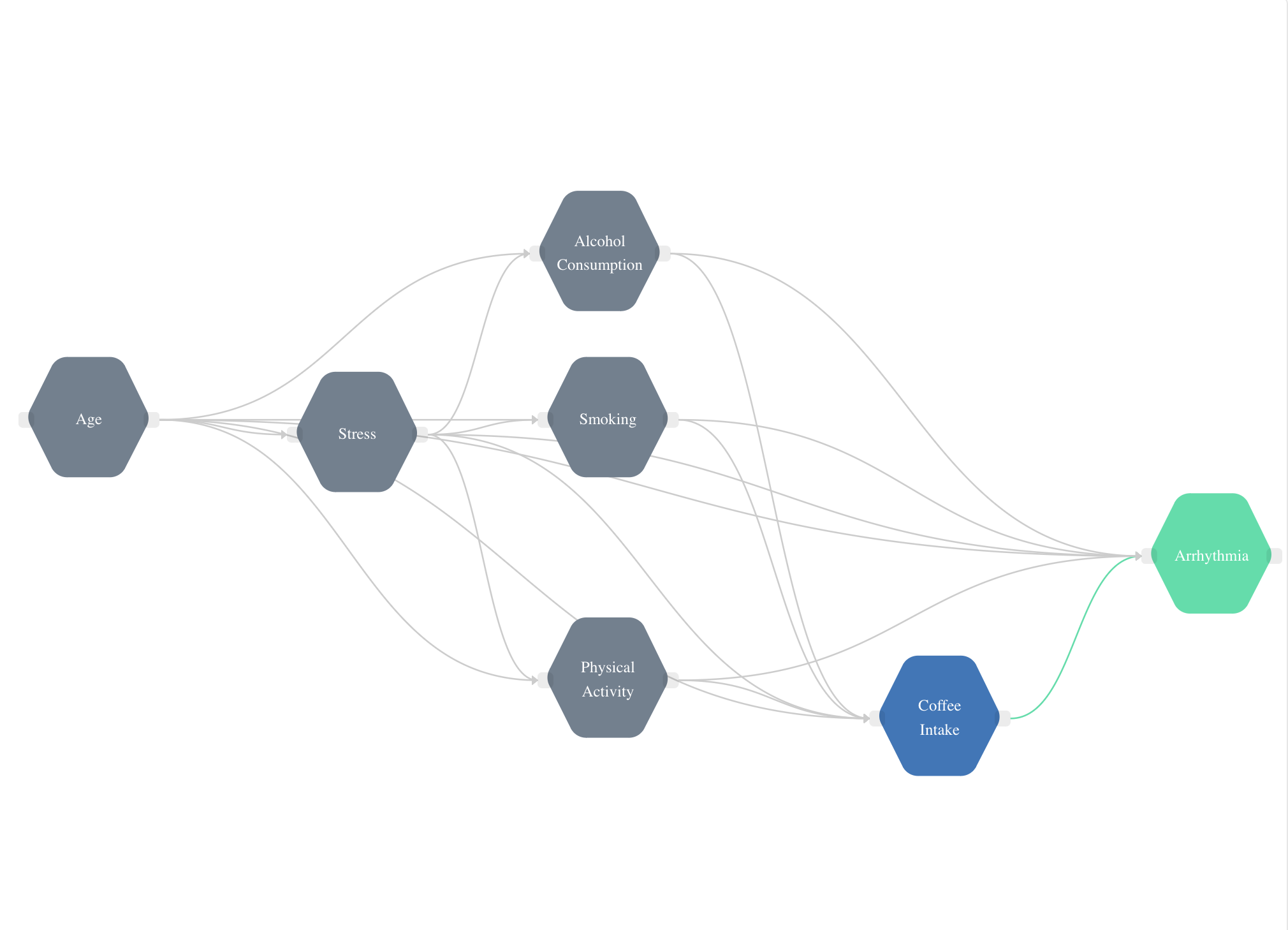
Resolution: From the graph, we need to adjust for the common causes in our model. After these adjustments, no further orange biasing paths are shown, and we get a valid estimate of the causal effect of coffee intake on arrythmias.
Causation via randomization
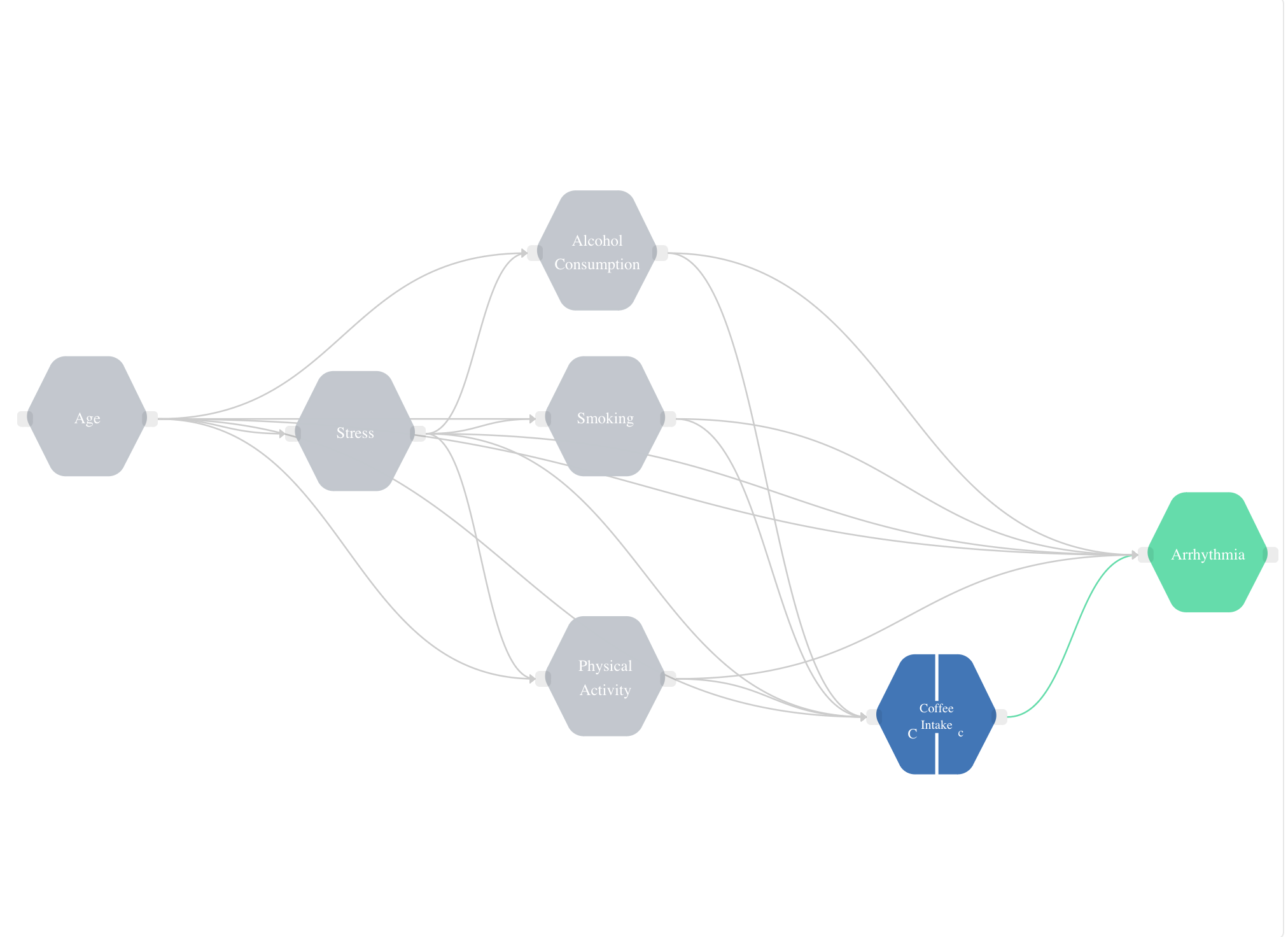
Alternative resolution: Instead of adjusting for the common causes in a regression model, if we are able to randomize coffee intake across a group of participants, we break the links to all causes of coffee intake. Then, the simple correlation between coffee intake and arrhythmia will be a causal estimate.
Randomization and correlation
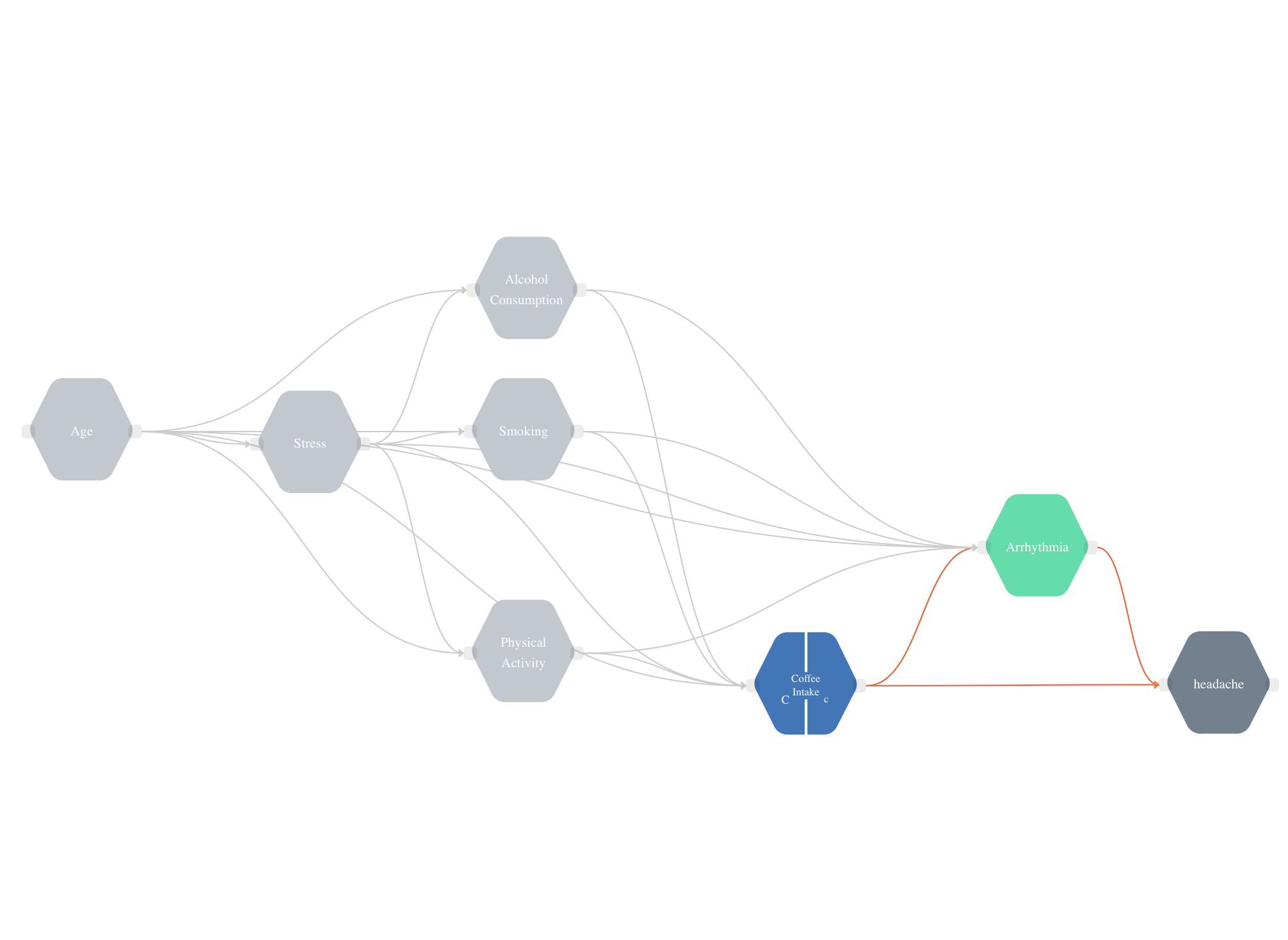
Setting: Headache incidence is a common effect of coffee intake and arrhythmias. When we adjust for this variable, we get an incorrect causal estimate because we introduce a biasing (orange) pathway. Note that randomization has not helped us here! What does the graph tell us? We learn from the graph that we should not adjust on headache incidence (common effect).
Randomization and correlation
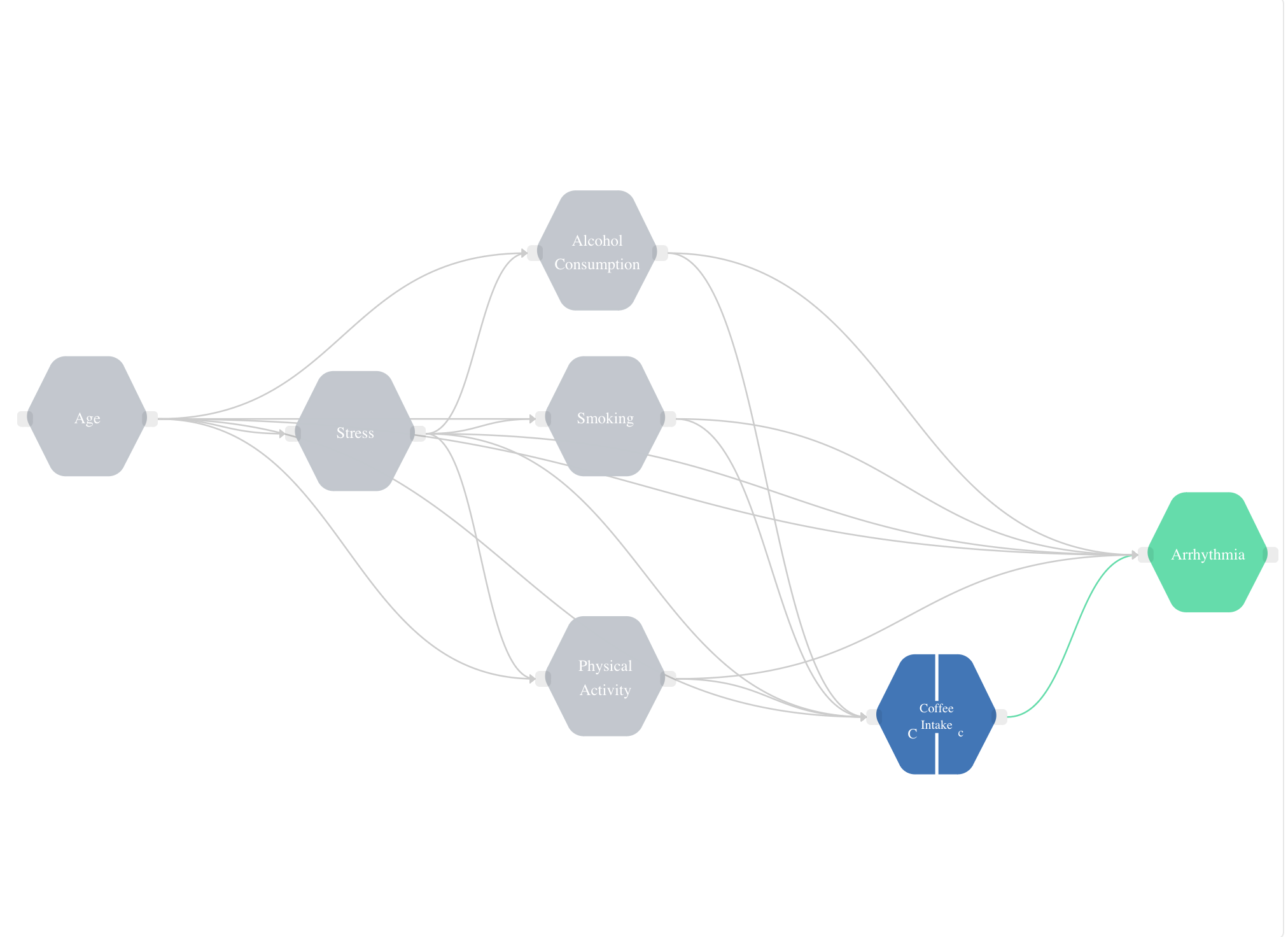
Resolution: As before, the simple correlation between coffee intake and arrhythmia is now a causal estimate.
State-of-the-art causal reasoning
cStructure empowers your team with a robust, efficient, and collaborative causal reasoning canvas.
Correlation does not imply Causation, See Why...
Low Code
Causal inference for everyone.
Empowering your entire research team to deliver stronger causal analyses — no coding required.
Collaborative
Real-time collaboration for instant team alignment.
Real-time team collaboration, version control, and integrated feedback loops.
AI Assistance
Kickstart and refine with generative AI knowledge assistants.
Generative AI, bias identification, and adjustment set optimization algorithms.
F.A.I.R. Projects
Store project data and metadata in the same schema.
Findable, Accessible, Interoperable, Reproducible causal analyses.
Trustworthy
Causal diagrams are transparent, rigorous, and precise.
Knowledge-driven structural causal models offer the transparency, rigor, and precision required for safety-critical industries.
JupyterLite
Privacy-preserving browser-based notebooks.
Rapid and private notebooks that can run in the browser or be transferred to your secure data and compute environment.
Meeting FDA's Draft RWE Guidance Requirements
The FDA's March 2024 draft guidance proposes expected requirements for non-interventional studies, emphasizing methodological rigor and validity.
Causal Inference Documentation Required
FDA now mandates sponsors provide a detailed approach to improve causal inference with methods that address confounding and bias (e.g. target trial emulation).
Mandatory Causal Diagrams
Study protocols should include causal diagrams that explicitly specify theorized causal relationships to support regulatory decision-making.
Confounding Factor Analysis
Sponsors should document specific approaches to account for potential confounding factors, including assessment of unmeasured confounding in non-interventional studies.
Variable Pathway Management
Studies should include evaluation of potential overadjustment of intermediate variables on the causal pathway to ensure methodological validity.
Causal Diagrams for Real World Evidence
Causality. Simplified.
Address regulatory requirements and strengthen your non-interventional studies with cStructure's collaborative causal diagram platform
Choose Your Causal Journey
Unlock the power of causal inference with a plan that grows with your needs
Causality. Simplified.
Get the help you need, when you need it
Our Mission
Deciphering the World's Complexity Together
Make Decisions with Confidence and Clarity
In a world overflowing with data, the challenge isn't just accessing information — it's understanding it. We don’t just provide a platform — we provide partnership. Our mission is to empower you to see the bigger picture, find the right answers, and make well-informed decisions based on clear, reliable causal insights.
cStructure
Public Beta 12.1.24 | Causality. Simplified.